I'm a machine learning researcher at Apple, working on multimodal AI.
Previously, I completed my Ph.D. in AI at
UC Berkeley in May 2024, co-advised by
Trevor
Darrell
and Joseph
Gonzalez
as part of the BAIR and Sky labs.
I was a visiting researcher at FAIR within Meta for 2 years during my Ph.D.
Before coming to Berkeley, I obtained my B.S. in computer science at Cornell University.
At Berkeley, I worked on improving the reliability of multimodal models, especially within
text generation. I focused on localizing and reducing hallucinations for vision + language models,
along with measuring and using uncertainty and mitigating bias.
Being in tech, I stereotypically enjoy climbing, hiking, skiing, running, and (basic) woodworking. In recent years, I've survived a
climbing accident, a nighttime bear encounter, and reviewer
2s. These have earned me metal screws in my ankle, a fear of Berkeley's mascot, and the papers below.
selected publications
-
An Introduction to Vision-Language Modeling
A great effort from Meta & collaborators, led by Florian Bordes! I am one of many co-authors.
arXiv 2024 -
ALOHa: A New Measure for Hallucination in Captioning Models
Suzanne Petryk*, David M. Chan*, Anish Kachinthaya, Haodi Zou, John Canny, Joseph E. Gonzalez, Trevor Darrell
NAACL 2024 (Oral) -
CLAIR:
Evaluating Image Captions with Large Language Models
David M. Chan, Suzanne Petryk, Joseph E. Gonzalez, Trevor Darrell, John Canny
EMNLP 2023 -
Simple
Token-Level Confidence Improves Caption Correctness
Suzanne Petryk, Spencer Whitehead, Joseph E. Gonzalez, Trevor Darrell, Anna Rohrbach, Marcus Rohrbach
WACV 2024, ICCV 2023 CLVL Workshop (Oral) -
Reliable Visual
Question Answering: Abstain Rather Than Answer Incorrectly
Spencer Whitehead*, Suzanne Petryk*, Vedaad Shakib, Joseph E. Gonzalez, Trevor Darrell, Anna Rohrbach, Marcus Rohrbach
ECCV 2022 -
On Guiding Visual Attention with Language
Specification
Suzanne Petryk*, Lisa Dunlap*, Keyan Nasseri, Joseph E. Gonzalez, Trevor Darrell, Anna Rohrbach
CVPR 2022 -
Remembering for
the Right Reasons: Explanations Reduce Catastrophic Forgetting
Sayna Ebrahimi, Suzanne Petryk, Akash Gokul, William Gan, Joseph E. Gonzalez, Marcus Rohrbach, Trevor Darrell
ICLR 2021
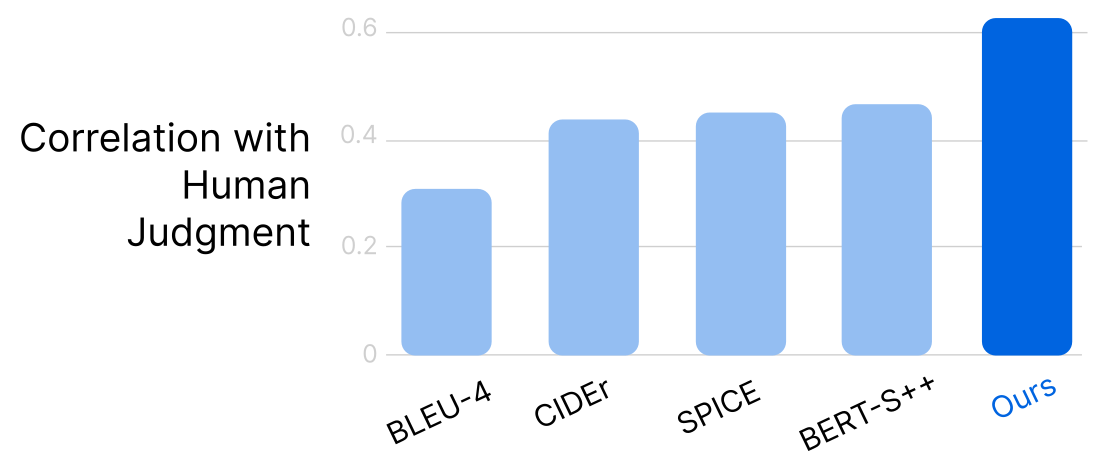
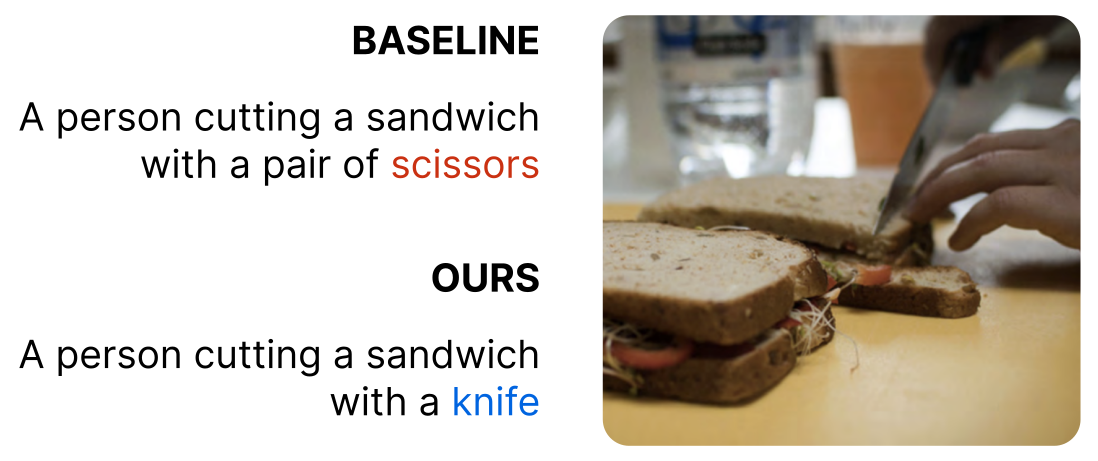
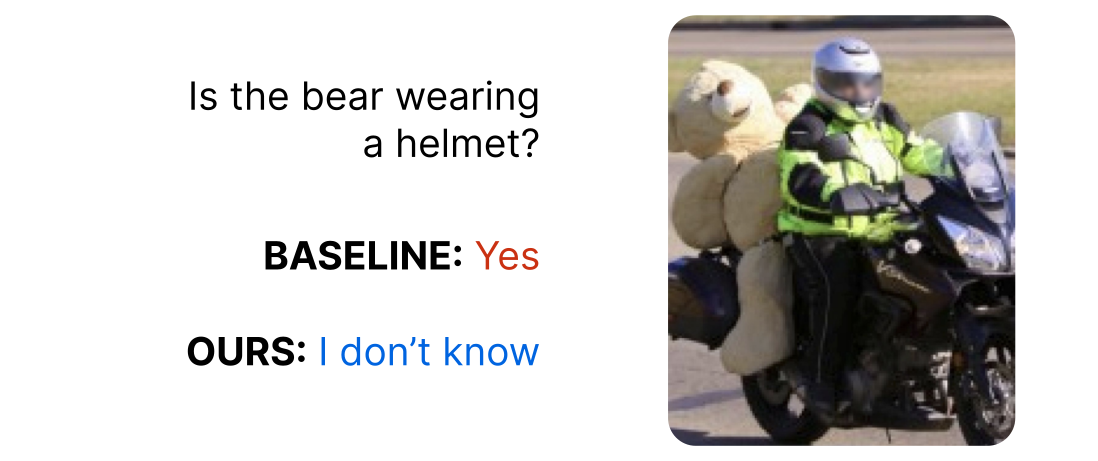
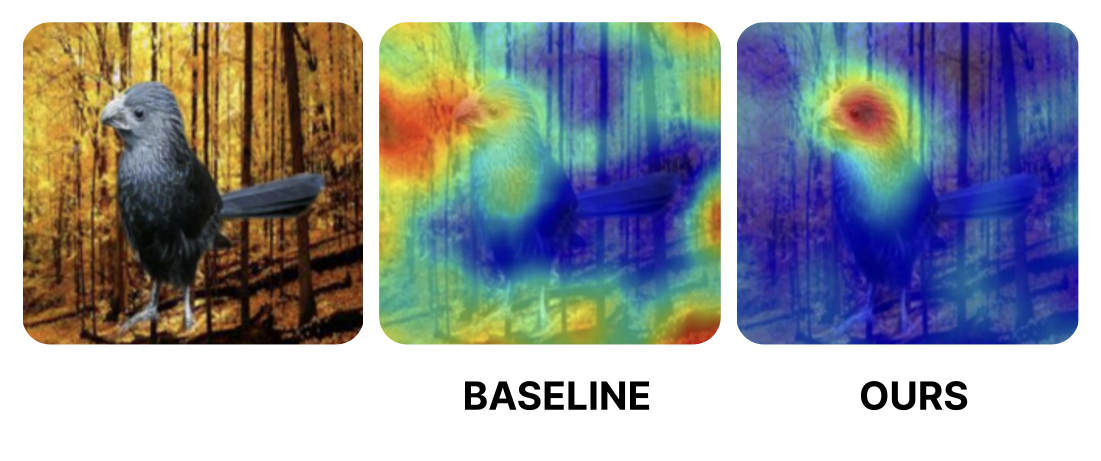